
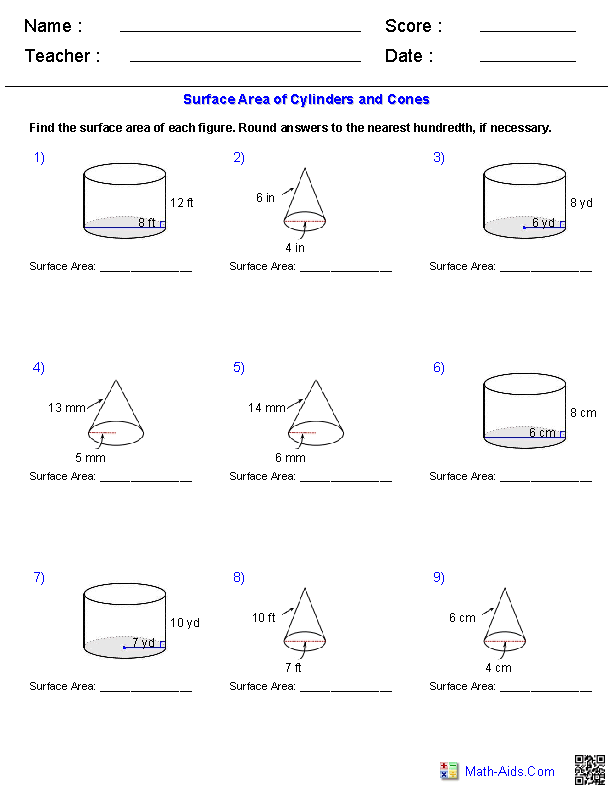
Specifically, we use (i) sparse generalized group additivity and (ii) kernel ridge regression as two representative classes of models, we propose a method combining rigorous model-based design of experiments and cheminformatics-based diversity-maximizing subset selection within the epsilon-greedy framework to systematically minimize the amount of data needed to train these models.
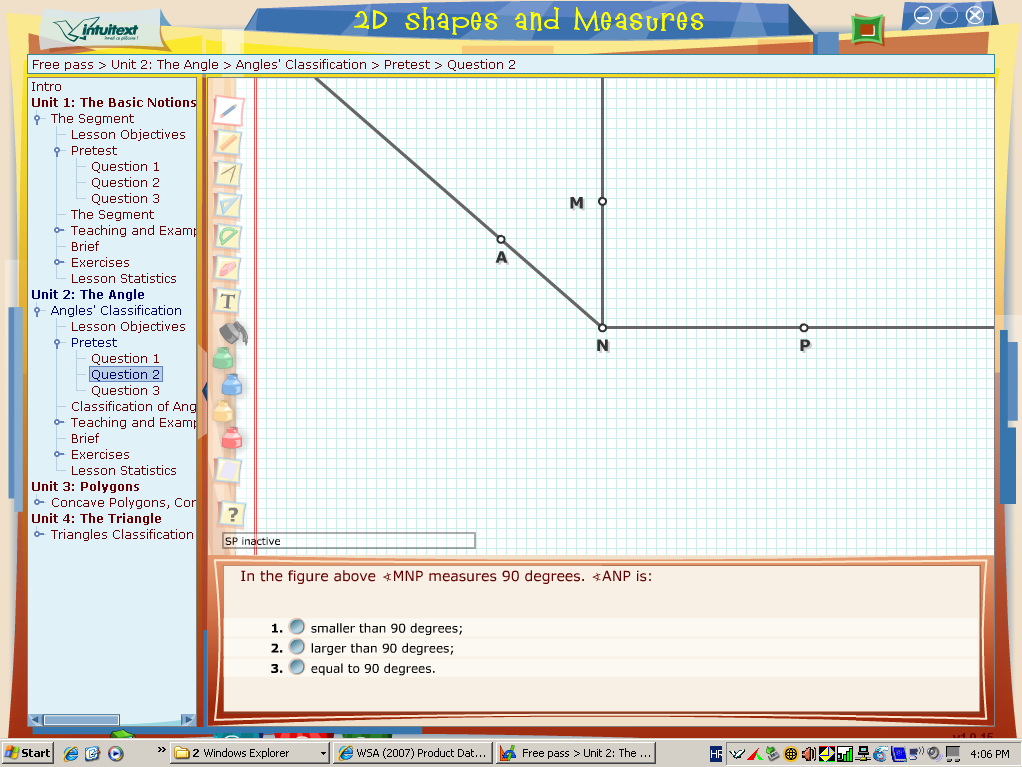
In this paper, we consider the problem of designing a training set using the most informative molecules from a specified library to build data-driven molecular property models. The method can exploit knowledge of the structural similarities between species and the consistency of the data to identify which species introduce the most error and recommend what future experiments and calculations should be considered.
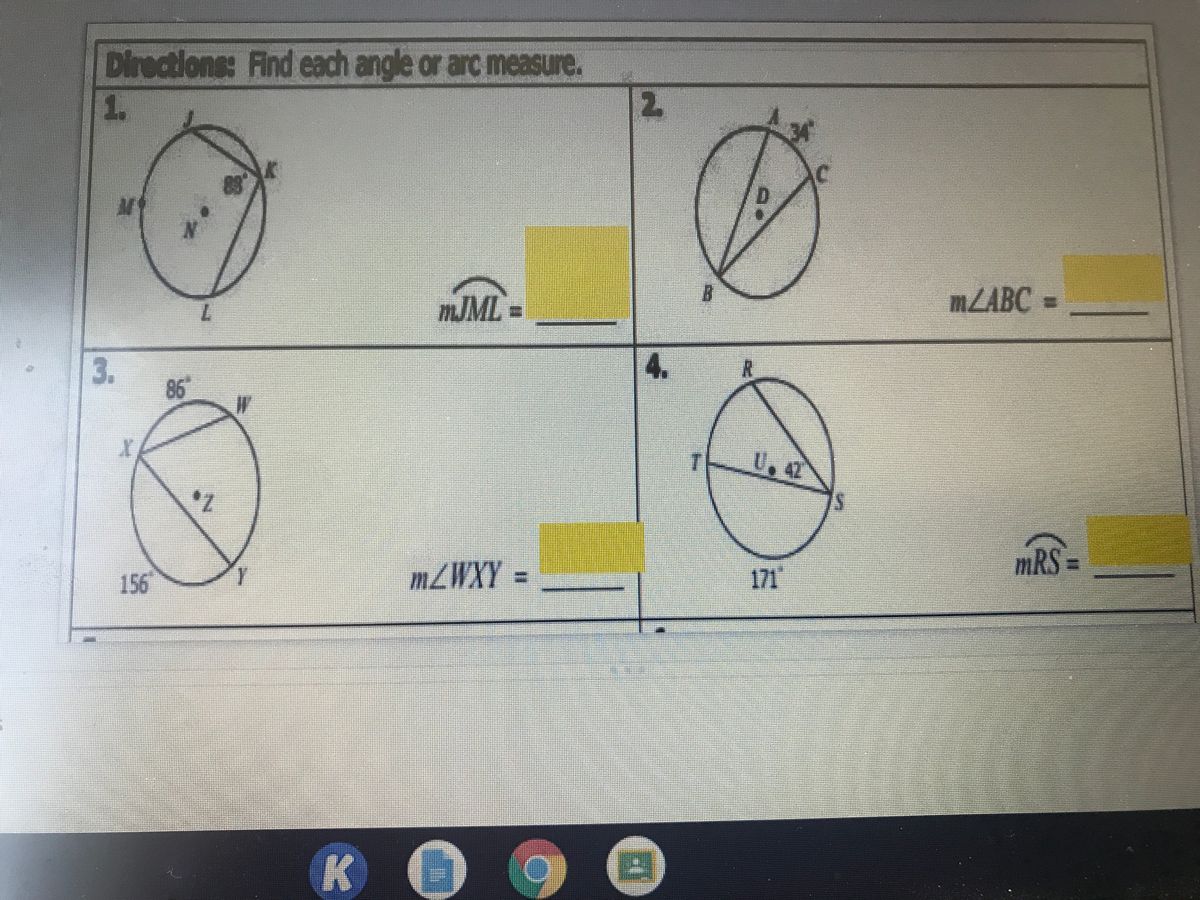
We find that this enables the rapid convergence of the calculations towards chemical accuracy. The method automates the identification and exclusion of inconsistent data. The concept of error-cancelling balanced reactions is used to calculate a distribution of possible values for the standard enthalpy of formation of each species. The framework is applied to validate data for the standard enthalpy of formation for 920 gas-phase species containing carbon, oxygen and hydrogen retrieved from the NIST Chemistry WebBook. We propose an automated framework to solve this problem by identifying which data are consistent and recommending what future experiments or calculations are required.
#Geometry find mjml 38 76 142 71 how to#
The challenge, however, is how to validate such large databases. The advent of large sets of chemical and thermodynamic data has enabled the rapid investigation of increasingly complex systems.
